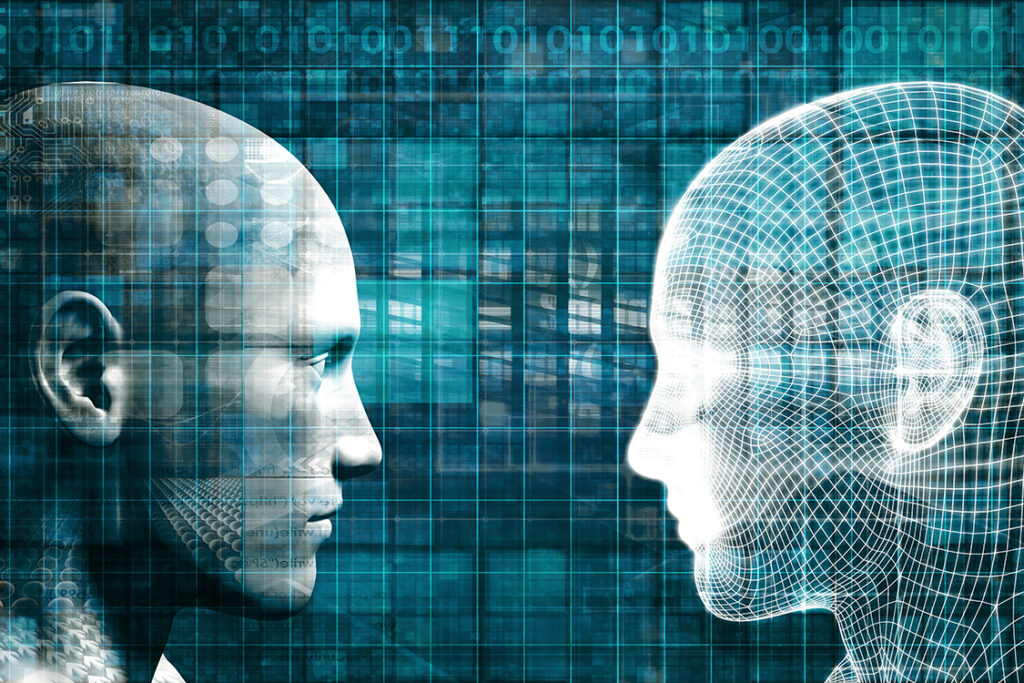
The UK, like every democracy in the world, faces increasing cybersecurity threats. The UK government is right to take action as these threats from state-sponsored adversaries and criminal groups continue to grow, annually. The government promises that Britain’s public services will be strengthened to further protect them from the risk of being shut down by hostile cyber threats.
The new strategy will be backed by £37.8 million to help local authorities boost their cyber resilience and will be implemented over the period of eight years from 2022 to 2030. Although the UK Cyber Security Strategy is a step in the right direction, especially with its emphasis on collecting events and identifying them before they become more serious incidents or breaches, eight years is far too long of a timeline. Why wait until 2030, when we can make far-reaching, impactful changes today?
Data and AI governance pitfalls
AI programmes require coordination, discipline, and organisational change, all of which become even more challenging with larger companies. In a small-to-medium enterprise that only has a few models in production, this may be far easier to manage in comparison to a larger organisation with thousands. What’s more, success is a question not just of processes, but a transformation of people and technology as well. This is why – despite the clear importance and tangible benefits of having an effective AI governance programme – there are several pitfalls that organisations can fall into:
A lack of senior sponsorship: AI governance programmes without senior sponsorship can lead to policies without any teeth. If there isn’t top-down castigation when AI governance policies aren’t being followed, it’s hard to adopt governance policies at scale.
Poor communication: A lack of communication around governance policies and standards can make an AI programme ineffective. Employees need to be made aware on a consistent basis of these and be advised on how they can implement them. In addition, companies need to be empowered with technology that allows knowledge-sharing and easy communication between teams building models and those conducting model risk analysis.
A lack of one central repository for AI models: Many organisations have disparate tools and techniques to manage their operationalisation when implementing AI at scale, increasing their risk factor exponentially.
The ingredients for a successful AI governance strategy
To overcome these pitfalls, companies must determine accountability by outlining who is responsible for each decision in the design as well as the deployment process across teams. This needs to be followed with intentionality by making sure that machine learning pipelines are aligned with organisational values. Finally, organisations must determine transparency by establishing how those pipelines are documented, and whether they are made explainable.
Establishing reliability of models and their operations as well as execution, and ensuring that they are governable and can be centrally controlled, managed and audited is, therefore, crucial.
Aligning AI governance with organisational ethics
Today, many companies that use data science have AI governance systems that have evolved semi-organically. It’s not uncommon to see several teams within a large group develop different AI systems, each using different technologies and data. Once deployed, models are monitored individually by their owners.
However, governance at scale means that projects must be monitored centrally. That means being able to see what data is used where at a glance, and how these models are performing. This enables AI to not only better spot model drift, but also identify models that are using questionable or risky datasets. This was the case of Amazon’s facial recognition algorithm, which had trouble recognising women and non-white people because white men were over-represented in the data used for its creation.
Addressing this risk is both human and technical. It is essential to educate teams developing AI systems, make them aware of the potential shortcomings and bear the responsibility for possible faults. Additionally, it is critical to recognise that empowering teams depends heavily on defining the ethics of the organisation first.
The bottom line
Ultimately, it is critical to ensure actions taken by AI systems respect ethical rules consistent with their environments. Organisations can develop their own AI frameworks with the right tools to ensure governance and a more ethical AI future.
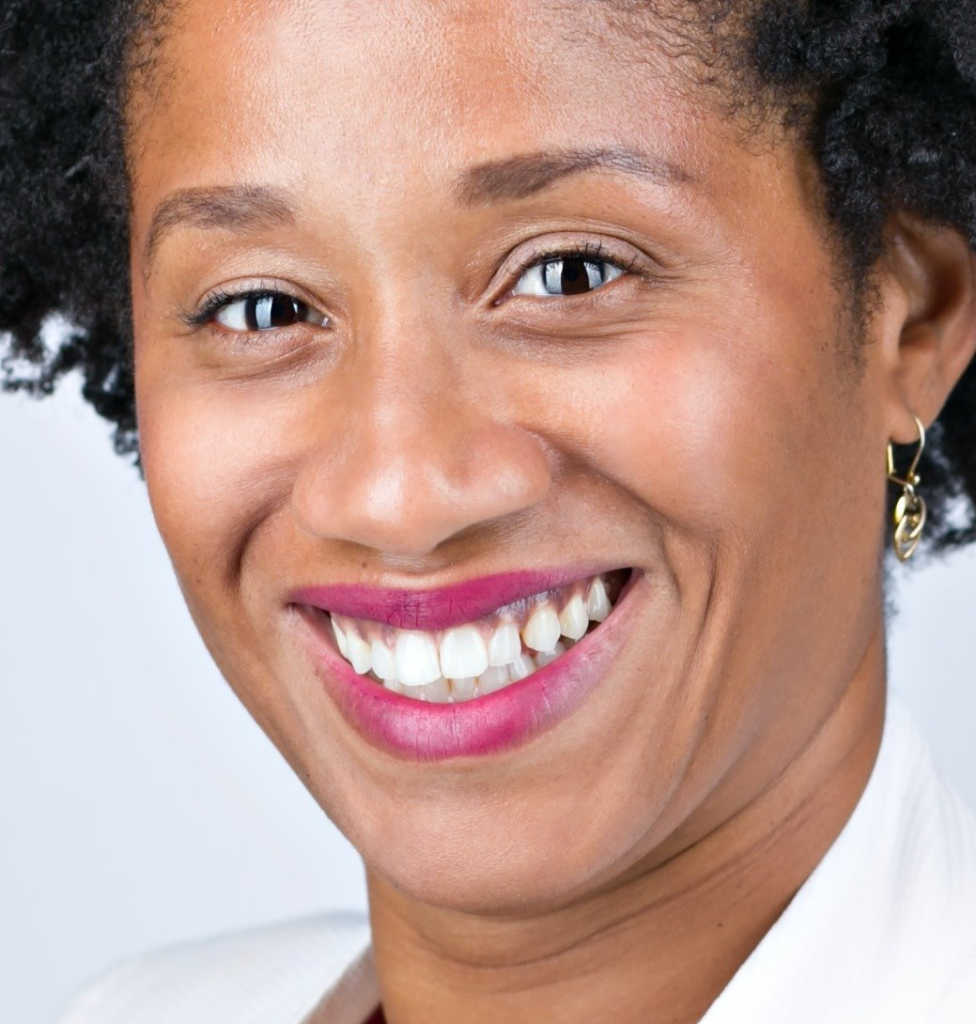
Simone Larsson is a prominent voice in the realm of AI and machine learning and an evangelist on the topic for Dataiku.