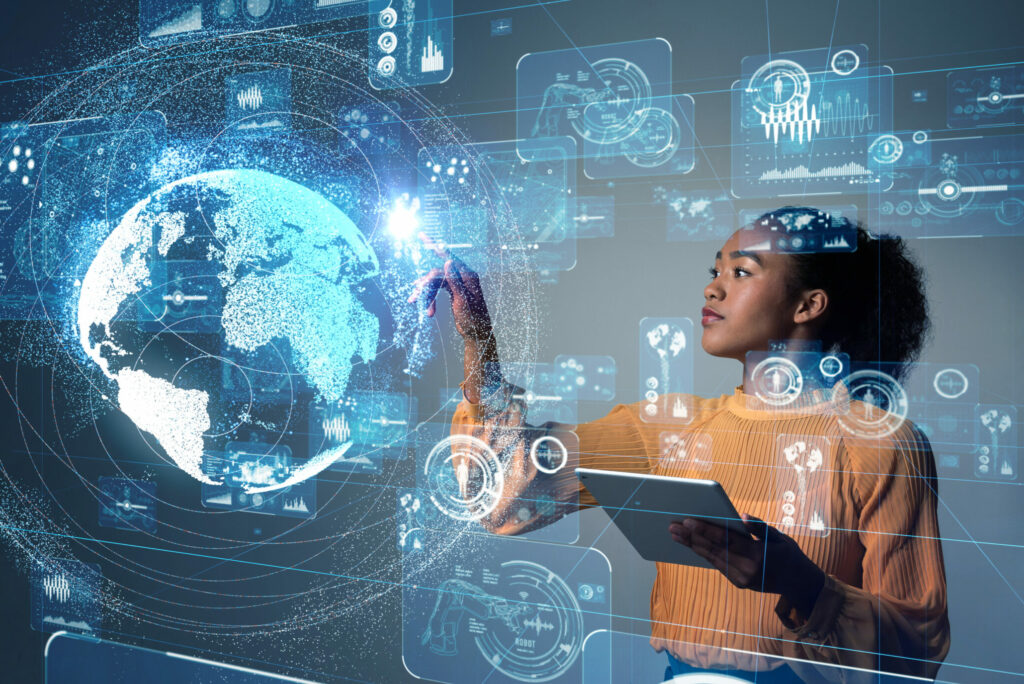
Inderpal Bhandari, Global Chief Data Officer of IBM, outlines the key elements needed for AI to become ‘trusted’ and gives his take on how it could revolutionise science in the future
There are few older heads in the data game than Inderpal Bhandari.
The Global Chief Data Officer of IBM was one of the four original pioneers of the CDO role globally and the only one of those still doing it. He became the very first CDO in the healthcare industry in 2006, and over the last 20 years has worked towards transforming industry-leading organisations and defining the scope, expectations, and deliverables of the modern CDO.
“I happened to be there at the right time and so I could grow with the profession,” says Bhandari, who is still recognised as one of the foremost authorities in the data and analytics space globally. “I’m the only one left of the original four, who is still working the job! I don’t know if that’s a good thing or a bad thing but it has allowed me to make a craft of it!”
And it’s not just the man himself. IBM, as a company, has been at the forefront of AI from the start. As much as a quarter of a century ago, the Deep Blue computer developed by the US multinational made headlines across the world when it won a chess game against world champion Garry Kasparov.
Now, some 25 years later, IBM remains at the forefront and is still investing massively in the space.
Following the $34 billion purchase of Red Hat a couple of years ago, the company continues to aggressively make acquisitions even now. BoxBoat Technologies and Bluetab have been bought in the past month or so alone and it’s clear to see that work in hybrid cloud, AI and data management are a massive strategic priority for IBM.
Bhandari is a huge believer in the power of AI. He spoke to Tech For Good about the importance of building trust in AI moving forward and what he considers to be the five core tenets in doing so. If these are met, he believes that AI has the potential to influence and reshape how the scientific method is employed.
“You have these central tenets of fairness, robustness, transparency, explainability, and privacy and security. That’s what makes up trusting data,” Bhandari says. “As you start infusing AI into key business processes, it starts absorbing the IT around those processes. An enterprise is then going to ask, ‘Can I really trust my IT provider here?’ So, that’s the first piece of trust that comes into play. It has to do with being essentially transparent, ethical. That trust is vital from an enterprise point of view. Why would you run the risk of having somebody disintermediate your business?
“You also have to consider the data that underlies the AI. How clean is it? Is it really fit for purpose? Are these models going to be run accurately? So now we get into the area of robustness. How robust is this system? Is it staying up to date as the data is being refreshed? Is it making sure that old data is getting weeded out? All those kinds of questions come up.
In terms of advancing the scientific method, there's a clear step and with the more complicated problems, AI becomes critical in dealing with them.”
“Another aspect of trust comes in when you’ve got somebody who’s actually making use of the AI system. If the recommendation is off the wall, and there’s no explanation and context to go with it, they won’t follow through on it. These days, the systems are powerful enough to analyse context but you’ve got to be able to explain the recommendation to the decision maker. That’s explainability, essentially.
“The final point is fairness. Are the recommendations fair for the people that are going to be impacted? So, if you’re doing recruiting, for instance, and the AI system makes a recommendation, are those or any recommendations actually going to be fair for the population at large? That is another aspect that becomes extremely important.”
With so many facets involved for AI to be successfully adopted, it becomes incredibly complex for enterprises to harness all that data and bring all these strands together.
IBM has realised that that’s the missing gap in the AI space: the fact there is no real platform there that essentially addresses trusted AI and gets you to trust it. The IBM Cloud Paks, built on Red Hat OpenShift, are AI-powered software solutions that can help organisations build, modernise, and manage applications securely across any cloud and were built with exactly that need in mind.
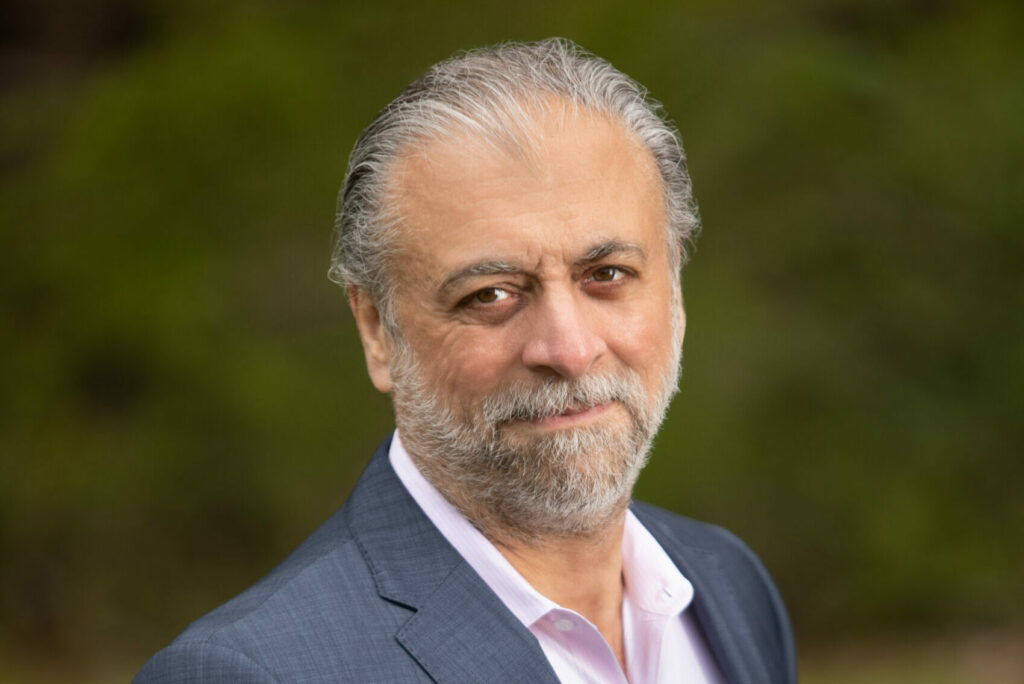
The most popular of these is the IBM Cloud Pak for Data, a unified platform delivering a data fabric to connect and access siloed data on-premises or across multiple clouds without having to move it. It simplifies access to data by automatically discovering and curating it to deliver actionable knowledge assets and also automates policy enforcement to safeguard use.
“In the five years my team has been using it, we’ve just seen huge strides,” Bhandari says. “The bottom line is it goes to addressing that complexity around those five core tenets and packages it in a way that the average enterprise can pick it up and run with it.”
An example of how this works practically for enterprises can be seen with IBM customer Regions Bank, which selected the company’s AI technology to help improve customer service and assist bankers in their everyday work.
The key challenge for IBM in working with Regions Bank was that the data they had was siloed all in all sorts of different places in a fragmented fashion. Using the Cloud Pak for Data platform simplified things for them, so that they could go from addressing the fragmentation, right to the models that were trusted and right through to being able to do AI.
“You’ve got data, you’ve got models, and then you’ve got processes,” Bhandari says. “There’s also the infusion of processes and you know, essentially how you’re going to infuse the AI into the process, how you are going to make sure that the humans in the loop in the process, are able to then work with AI and so forth?
“We appreciated very quickly that this is the gap in terms of what enterprises are missing. There are also difficulties that have to do with infusing AI into processes that make it more challenging and difficult. The Cloud Pak for Data and some of the other Cloud Paks [there are currently six Cloud Paks: Data; Business Automation; Watson AIOps; Integration; Network Automation; and Security] that we came up with, they are meant to address that totality.
“We’ve also invested and acquired more quite recently, over the last year or so, several companies that play in the space of different domains, different verticals, and they essentially understand those verticals very, very well.”
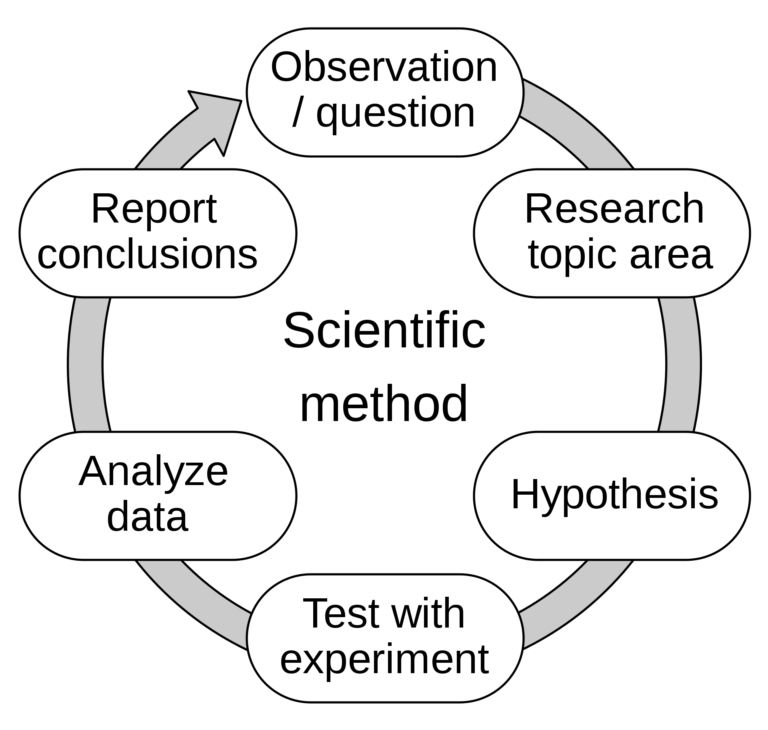
Investing in companies which understand these other verticals and regions is massive for IBM but they are also building these verticals into their own cloud platform.
In their cloud for finance and banking, they’ve ensured that industry regulations have been built into the ecosystem to make it more useful for end-users, and to allow them to start making use of it immediately. But, in Bhandari’s eyes, the potential for this goes much further than just helping customers to manage their data and automate their processes. He can already see evidence of how their work is helping advance science and dealing with some of the world’s biggest and most pressing problems.
IBM and AI
IBM believes in augmented intelligence “with a human remaining in the loop” and that the purpose of AI and cognitive systems developed and applied by the company is to augment – not replace – human intelligence.
In the company’s view, moving forward, “build for performance” will not suffice as an AI design paradigm and that we must learn how to build, evaluate and monitor for trust. With that in mind, IBM Research AI is developing diverse approaches for how to achieve fairness, robustness, explainability, accountability, value alignment, and how to integrate them throughout the entire lifecycle of an AI application.
“After the outbreak of COVID-19, we set up a high-performance computing consortium, which scientists could use from across the world to help in the response to the pandemic,” he explains. “A lot of that had to do with essentially how viruses and other molecules interact. There are literally billions and billions of events that take place, and we have to be able to model out a simulation like that.
“We put together this high-performance computing consortium where a number of companies came together, and we allowed them to essentially have access to our supercomputer to be able to do this. So, there’s a whole science community that came together around that, and then went through the scientific method to be able to do it.”
Bhandari’s excitement stretches beyond that, though. He believes that the same scientific method itself could even change as a result of AI becoming more pervasive and more trusted.
“In terms of advancing the scientific method, right, there’s a clear step,” he says. “It’s a sequenced method where you observe some stuff, you come up with a hypothesis, you test your, say, five hypotheses, and that loop goes on ad infinitum as you as you start testing it out, and so forth. And with the more complicated problems, AI becomes critical in dealing with them.
“We talked about how AI can assess the quality of the data and whether it’s fit for purpose. With regard to coming up with a hypothesis and to be able to then test that out, again, there’s tremendous applicability for AI. At IBM Research we have a program called IBM Project Debater that comes up with its own hypotheses in response to what the other team is saying, but you can see that there’s a whole potential there for applying AI to the hypothesis-generation aspect.
“Then, similarly, for the refinement and the testing, you’re able to apply AI at every step. So, I think that the scientific method would be greatly accelerated as we go forward with AI into the future, and especially trusted AI.”